Kabir Aladin Verchand
verchand [at] usc.edu
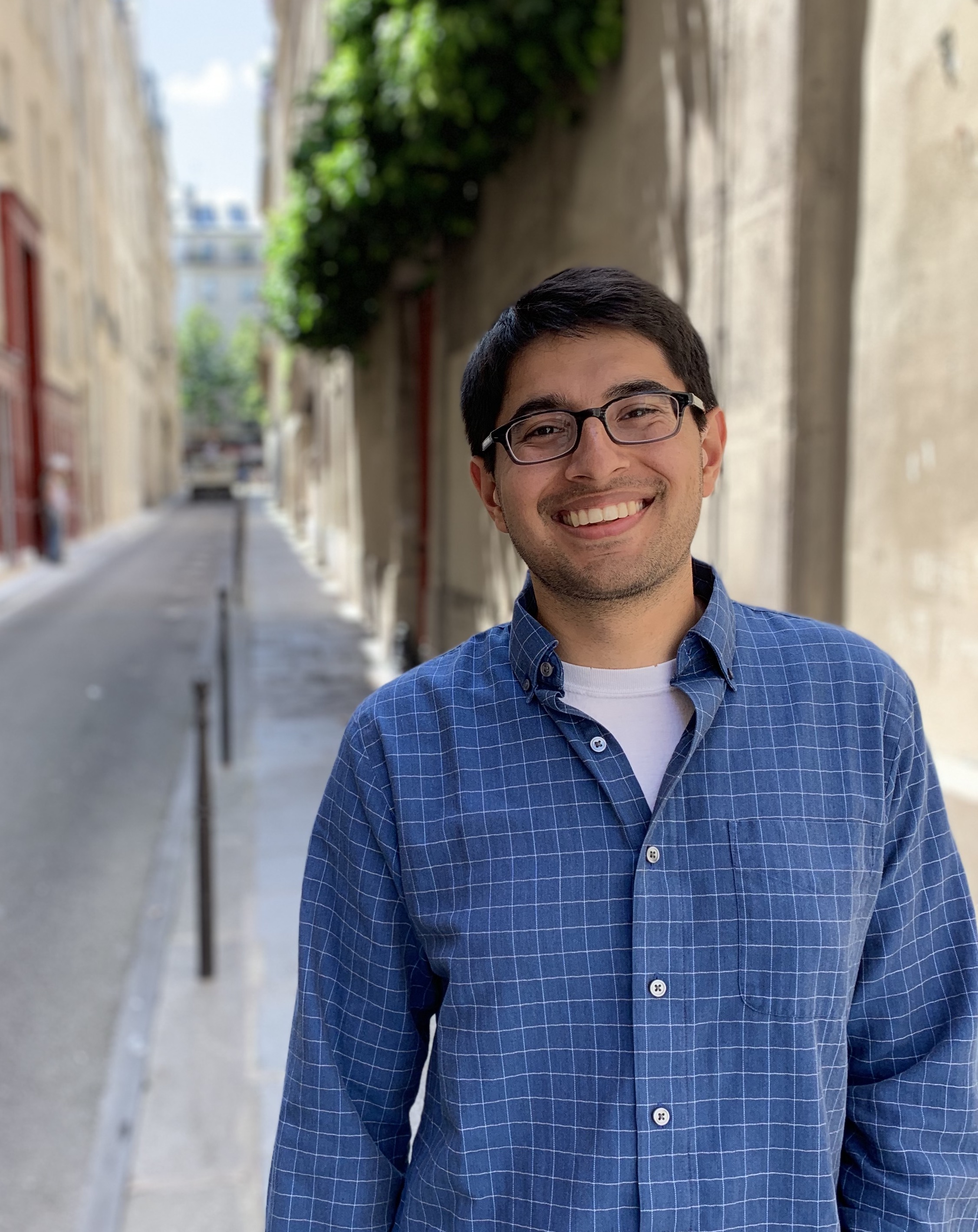
I am a postdoctoral scholar hosted jointly by Richard Samworth at the Statistical Laboratory, University of Cambridge and Ashwin Pananjady at the Schools of Industrial and Systems Engineering and Electrical and Computer Engineering, Georgia Tech, where I am the Gary C. Butler Family Postdoctoral Fellow. Previously, I obtained my PhD in Electrical Engineering at Stanford University, where I was advised by Andrea Montanari.
I am broadly interested in problems at the intersection of optimization, statistics, and computational complexity. In particular, I am interested in understanding both statistical and computational aspects of learning from random (as opposed to worst case) data.
Note: I previously published under the name Kabir Aladin Chandrasekher.
In Fall 2025, I will join the University of Southern California (USC) as an assistant professor in the Department of Data Sciences and Operations
Selected recent publications (see all)
- Chandrasekher, K.A., Pananjady, A., and Thrampoulidis, C. (2023), Sharp global convergence guarantees for iterative nonconvex optimization: A Gaussian process perspective, Annals of Statistics.
- Runner-up: Best paper prize for young researchers in continuous optimization, Mathematical Optimization Society
- Ma, T., Verchand, K.A., Berrett, T.B., Wang, T., and Samworth, R.J. (2024), Estimation beyond Missing (Completely) at Random, (preprint).
- Mardia, J., Verchand, K.A., and Wein, A.S. (2024), Low-degree phase transitions for detecting a planted clique in sublinear time, Conference on Learning Theory (COLT).